Data is the foundation for effective decision-making in today's business environment. Accurate data analysis makes it possible to optimise processes, manage the inventory in a better way, and forecast demand or personalise customer offers. However, even top-of-the-range IT tools without the ‘fuel’ of cleansed data will not protect us from huge financial losses.
Key findings of the article
- Poor data quality leads to faulty strategies and drastically increases operational costs.
- Cleansing data is the first step to take; it provides the basis for more accurate and effective business decisions.
- Companies that prioritise the quality of their data record realise significantly higher profits through increased productivity and customer satisfaction.
Millions in losses you can avoid!
Maintaining distributed data comes at a cost. Time and resources are wasted while processing data manually, searching for and correcting errors, and re-entering information, which raises the risk of serious human error and affects business productivity. Your team could spend this time on more important tasks.
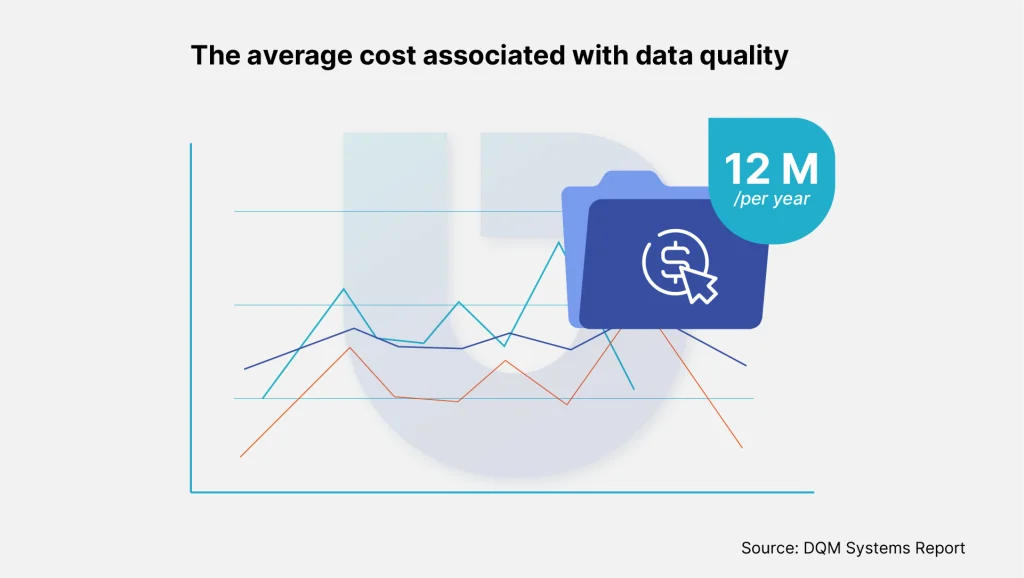
Indirect costs are even more acute. Incorrect data in the retail industry leads to mismanagement of stock – resulting in stock shortages at crucial times or overstocking during the off-season.
Walmart – a major retail chain – experienced serious inventory management problems due to outdated or inaccurate data over the festive period, resulting in stock shortages in high-traffic shops and overstocking in less busy locations. This resulted in customer dissatisfaction and a significant loss of revenue. In turn, excess stock had to be discounted or disposed of, with further affecting profit margins. In logistics, inaccurate data on delivery routes means higher fuel costs or increased delivery times.
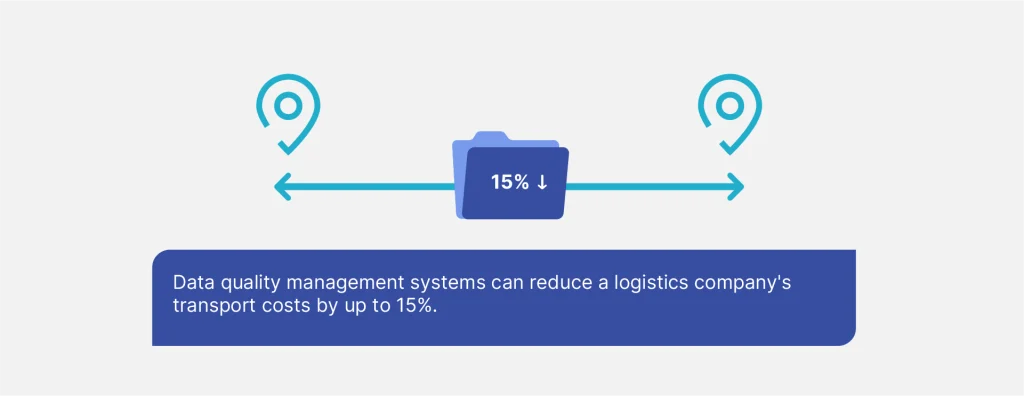
Eliminate costly mistakes
By addressing these challenges head-on, you can eliminate costly mistakes and boost the efficiency. Did you know that harnessing the full potential hidden in your data allows you – for example – to increase sales results and create more effective advertising campaigns? Here are some basic ways to avoid problems with poor quality data you have.
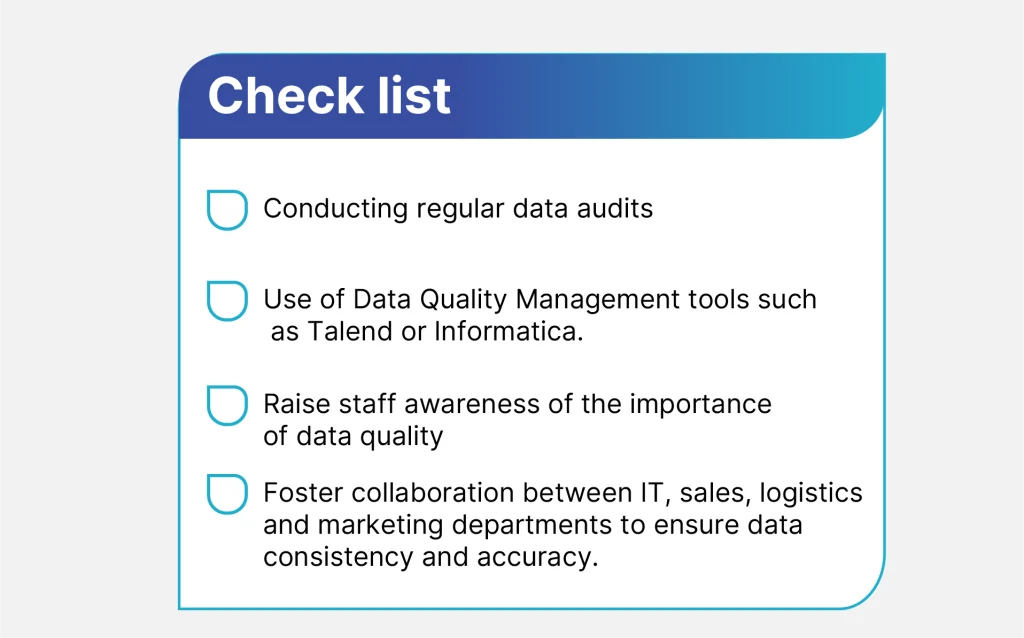
Turn your data into profits – best practice in data quality assurance
Now that we have seen how to prevent mistakes, let us explore how to achieve a clean database. Higher data quality paves the way to higher profits. Find out what other practices you can implement in your business to keep your data in top condition and reap maximum benefits.
- Data governance structure – implement a comprehensive data governance framework and create clear rules, roles and responsibilities for data management.
- Data integration and consolidation – use data integration tools to create one consistent source of knowledge with easy access across the organisation.
- Real-time data processing – invest in real-time data processing technology to detect and correct data quality issues immediately.
- Training and education – provide training on data management best practices to your staff, enabling them to benefit from the full potential of tools and processes.
- Automated data validation – introduce automated data validation processes in your business to check for anomalies, inconsistencies and errors at an early stage of data processing.
- Data quality indicators and KPIs – develop and monitor key performance indicators (KPIs) to assess the effectiveness of initiatives undertaken and identify areas for improvement.
- Data cleansing initiatives – initiate data cleansing projects to remove duplicated, outdated and incorrect data from databases, thus preventing problems resulting from data corruption.
- Feedback mechanisms – ensure that feedback mechanisms are implemented so that users can report data problems and suggest improvements.
A clean database – what is the return on investment?
It is clear, that investing in data quality brings significant benefits, increasing decision-making accuracy and operational efficiency. Clean data allows more accurate demand forecasting, inventory optimisation and more effective route planning in logistics. This enables companies to reduce costs, improve sales performance and increase customer satisfaction. In addition, precise data supports the creation of effective marketing campaigns, reduces error costs and minimises regulatory risks.
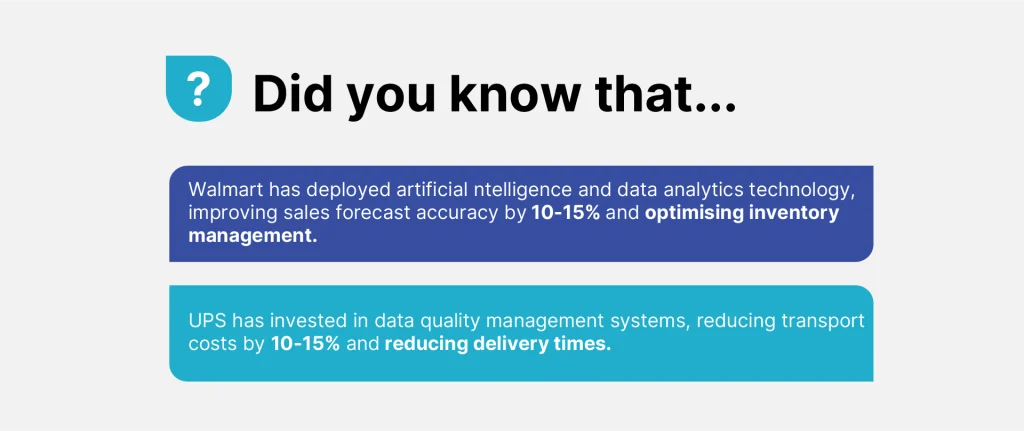
Conclusion
Cleaned and structured data plays a key role in making sound decisions. Business leaders in the retail and logistics sectors should prioritise data quality – both as a strategic investment that will drive growth and competitive advantage as well as reduce the risk of serious financial loss. By implementing best practices, using the right tools and fostering a data-driven culture, organisations can significantly improve their operational efficiency, customer satisfaction and overall business performance.